Why Predictive Maintenance Is the Future of Industrial Iot
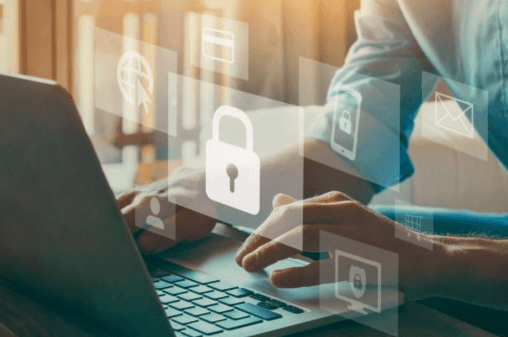
As industries increasingly rely on connected devices, the necessity for efficient asset management has never been more critical. Predictive maintenance stands out as a transformative approach within the Industrial IoT landscape, leveraging advanced data analytics and machine learning to foresee equipment failures. This proactive strategy not only enhances operational efficiency but also significantly impacts cost management and asset longevity. However, the implications of adopting such technologies extend beyond mere maintenance practices, raising questions about their broader role in shaping future industrial operations. What might this evolution look like in practice?
Understanding Predictive Maintenance
Predictive maintenance (PdM) is a proactive approach to equipment maintenance that leverages data analytics and IoT technology to anticipate failures before they occur.
By employing sensor integration and sophisticated equipment monitoring, PdM facilitates accurate failure prediction and optimized maintenance scheduling.
This strategy not only promotes operational efficiency but also drives cost reduction and downtime minimization, ensuring an agile and responsive industrial environment.
Key Benefits for Industries
Implementing predictive maintenance in industrial settings brings forth a multitude of benefits that significantly enhance operational performance.
Key advantages include cost reduction through minimized downtime, extended equipment lifespan, and improved asset optimization.
Leveraging data analytics enhances operational efficiency and risk management, while fostering workforce productivity.
Ultimately, predictive maintenance empowers industries to achieve a proactive stance, ensuring seamless operations and superior resource utilization.
Technologies Driving Adoption
At the forefront of predictive maintenance adoption in industrial IoT are several key technologies that facilitate data-driven decision-making and operational efficiency.
Machine learning and predictive algorithms analyze data collected through advanced sensor technology, while data analytics provides actionable insights.
Cloud computing and edge computing enhance real-time monitoring capabilities, allowing IoT platforms to integrate seamlessly, ultimately driving improved maintenance practices across industries.
Future Trends in Predictive Maintenance
As industries increasingly leverage the technologies driving predictive maintenance, the future of this discipline is poised for significant transformation.
Enhanced data analytics and machine learning will optimize predictive algorithms, enabling real-time monitoring and improved sensor integration.
This evolution will drive cost reduction and bolster operational efficiency, transforming asset management practices and paving the way for a more proactive, data-driven approach to maintenance strategies.
Conclusion
In contrast to traditional maintenance strategies that react to failures, predictive maintenance embodies a proactive approach, leveraging real-time data analytics to foresee equipment issues. This paradigm shift not only enhances operational efficiency but also significantly reduces costs associated with unplanned downtimes. As industries increasingly adopt advanced technologies, the future of predictive maintenance promises a more resilient and agile operational framework, ultimately redefining asset management and paving the way for a data-driven industrial landscape.